I do enjoy talking to customers and discuss the challenges they are facing in the system, services, limitations etc. I may not be solving their architectural challenges instantaneously, but I would sleep on it to get the most optimized solution for their business model. (Remember – “Optimized” will vary based on the actual needs and depends on customer)
Key challenges of any business with conventional data processing system.
- Most of the conventional system process the data in batches (hourly(s), nightly, weekly) which is considerably late for most of the business in the current competitive market.
- To get the real-time feedback about your business/products from your end users/consumers.
To address the above challenges, I am demonstrating how we can make use of modern technologies to process the streaming data to make better sense of the data.
Note: Any data landing on a platform or in a format which is unfriendly to analyze are no better than having no data.
Architectural diagram to handle real-time data.
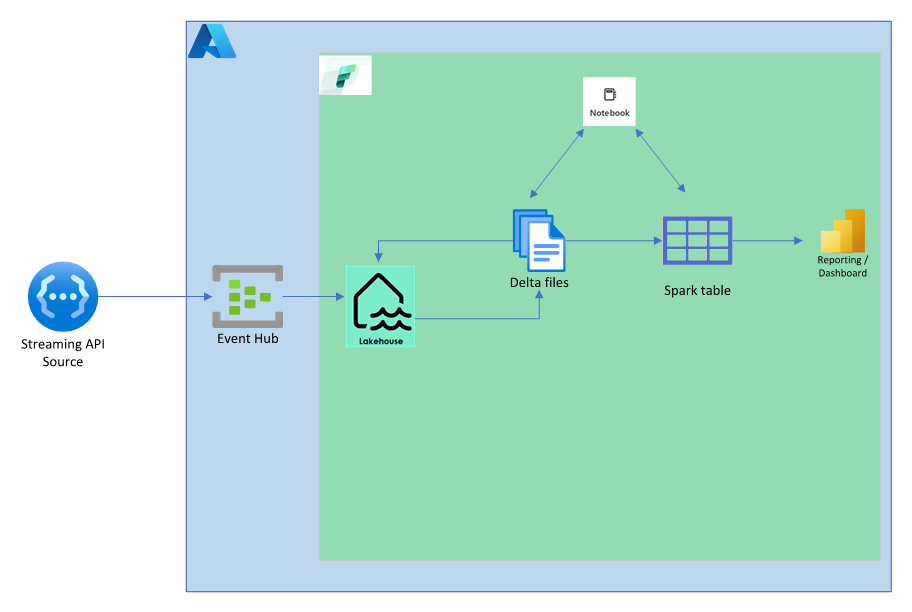
- For the purpose of demonstration, we have used “coin cap” open streaming API which gives real time pricing of crypto currency, (focused on bitcoin price).
- Using Azure event hub as the first landing zone to stream the data to second landing zone which is the latest Microsoft Fabric.
- Spark cluster in Microsoft Fabric has been used to process and pick the relevant data which is essential for business insights.
Tip: You can use twitter or any social networking API to retrieve the data related to your product or service and understand the consumer feedback much faster and prevent brand name damage. Perhaps tracking product hash tag would be a better pick to start with.
Pre-requisites:
- Python platform to make API calls. This is my streaming application.
- Azure event hub for stream and ingestion. If you don’t have one, get it created referring What is Azure Event Hubs? – a Big Data ingestion service – Azure Event Hubs | Microsoft Learn
- Create Microsoft Fabric and get familiar with the user interface. Data Analytics | Microsoft Fabric
Step 1: Set up the streaming python application. (Source)
Note: Here I am setting up a python application to stream the bitcoin Realtime price every second. EVENT_HUB_CONNECTION_STR = You get the connection string from Event hub namespace -> Shared Access Policies -> Properties of the policy. EVENT_HUB_NAME = event hub name, not the name space.
<code>
import asyncio
import requests
from azure.eventhub import EventData
from azure.eventhub.aio import EventHubProducerClient
EVENT_HUB_CONNECTION_STR = "<your event hub connection string>"
EVENT_HUB_NAME = "<your event hub name>"
async def run():
while True:
await asyncio.sleep(1)
producer = EventHubProducerClient.from_connection_string(conn_str=EVENT_HUB_CONNECTION_STR, eventhub_name=EVENT_HUB_NAME)
async with producer:
# Create a batch.
event_data_batch = await producer.create_batch()
url = "https://api.coincap.io/v2/rates/bitcoin"
payload={}
headers = {}
response = requests.request("GET", url, headers=headers, data=payload)
data=(response.json())
event_data_batch.add(EventData(str(data)))
# Send the batch of events to the event hub.
await producer.send_batch(event_data_batch)
# A tracer message which shows the event interval
print("sent to azure successfully")
loop = asyncio.get_event_loop()
try:
asyncio.run(run())
loop.run_forever()
except KeyboardInterrupt:
pass
finally:
print("Closing the loop")
loop.close()
Step 2: Setup up the Lakehouse in fabric
- Login to the Microsoft fabric account and create a Lakehouse.
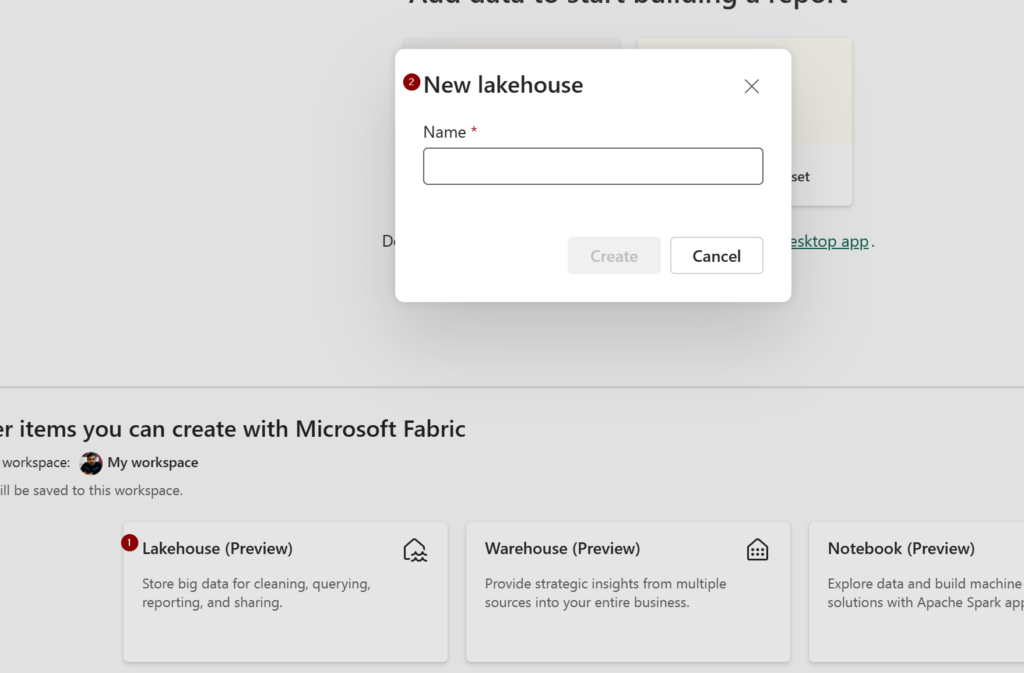
- Save the path and URL of both tables and files (from properties). This is needed in our query to create delta late and spark tables respectively.
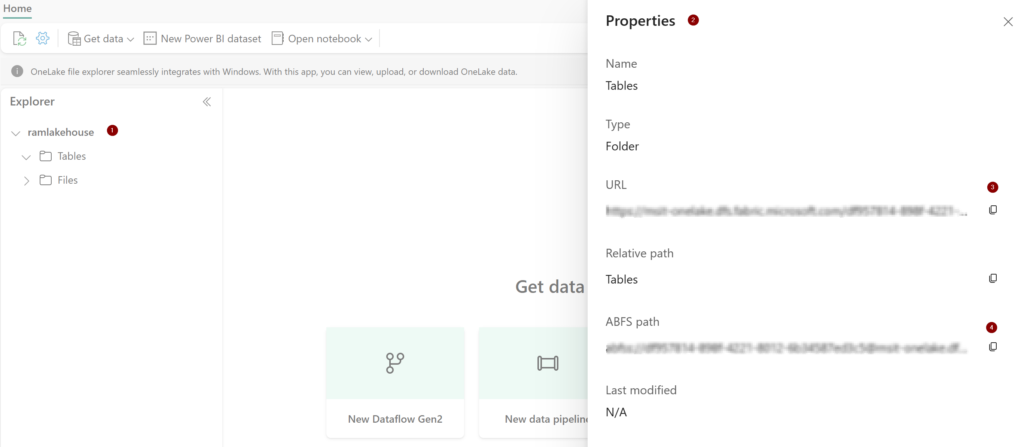
Tip: You can also download OneLake explorer app if you prefer Download OneLake file explorer from Official Microsoft Download Center.
Step 3: Code to consume the data from event hub and store it in a DeltaLake.
- Use your event hub connection string, make sure that you have minimum read permission.
- Define the streaming content.
<code>
from datetime import date
ehConf = {
'eventhubs.connectionString' : sc._jvm.org.apache.spark.eventhubs.EventHubsUtils.encrypt('<your event hub connection string with shared access key>')
}
df_streaming = spark.readStream.format("eventhubs").options(**ehConf).load()
df = df_streaming.withColumn("body", df_streaming["body"].cast("string"))
- Define the delta lake.
- Files/DeltaLake/Delta_Tables/ -> Create subdirectories inside your OneLake “Files”. This is where you delta table is going to be.
- “checkpointLocation”, -> This is my another dierctory inside files to store checkpoint files and other metadata.
- Little modification in file path just to partition the data every day, this will give is the flexibility of choosing the subset.
<code>
DeltaLakePath=("Files/DeltaLake/Delta_Tables/"+date.today().strftime("%Y%m%d"))
df.writeStream \
.format("Delta") \
.outputMode("append") \
.option("checkpointLocation","abfss://<abfss connection string saved earlier from one lake>") \
.start(DeltaLakePath)
- Define spark table from delta for analysis/reporting.
- Spark table will be created for each day of data.
<code>
DeltaLakePath=("Files/DeltaLake/Delta_Tables/"+date.today().strftime("%Y%m%d"))
defTable='CREATE TABLE bitCoin_'+date.today().strftime("%Y%m%d")+' USING DELTA Location '+"'"+DeltaLakePath+"'"
spark.sql(defTable)
Tip: Yes, I have the same copy of data in spark table now, its redundant data!!! Pick one on your convenience.
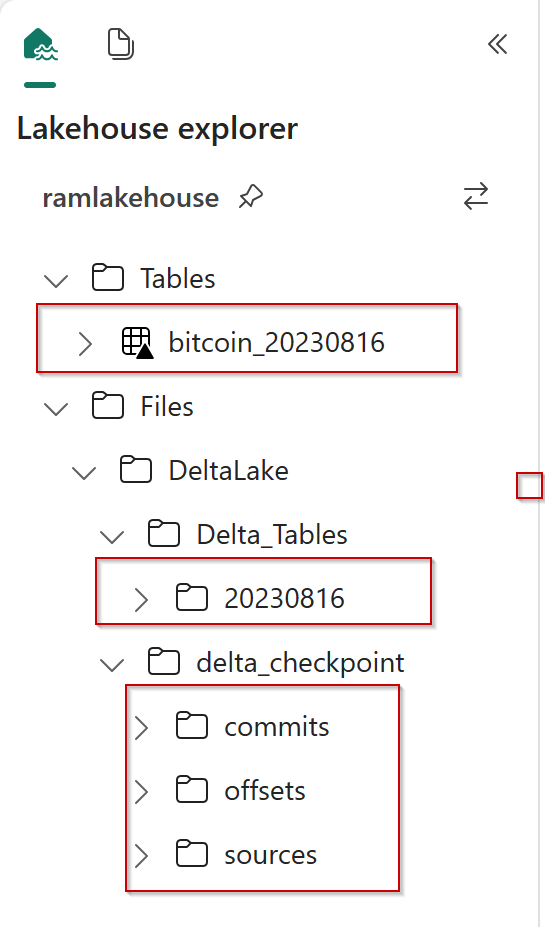
Let us check the table data via explorer.
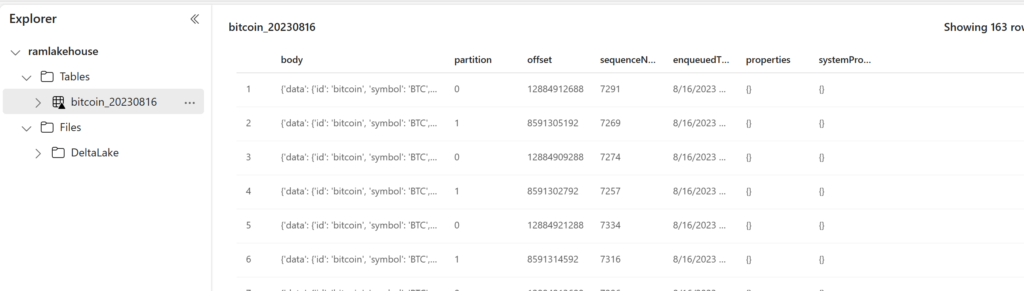
Let us check the Delta Lake data.
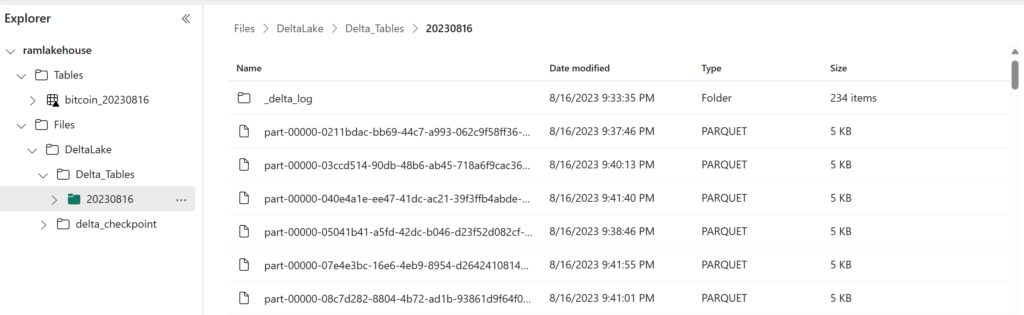
Great !! files are loaded. Now we have to deal with json and process further to clean the data.
json we have -> {‘data’: {‘id’: ‘bitcoin’, ‘symbol’: ‘BTC’, ‘currencySymbol’: ‘₿’, ‘type’: ‘crypto’, ‘rateUsd’: ‘29017.4373609315518503’}, ‘timestamp’: 1692218138650}
For this demo focus are on crypto name, price and time. Hence thought of a view for picking what I want.

There you go!!! We have the real-time bitcoin price now.
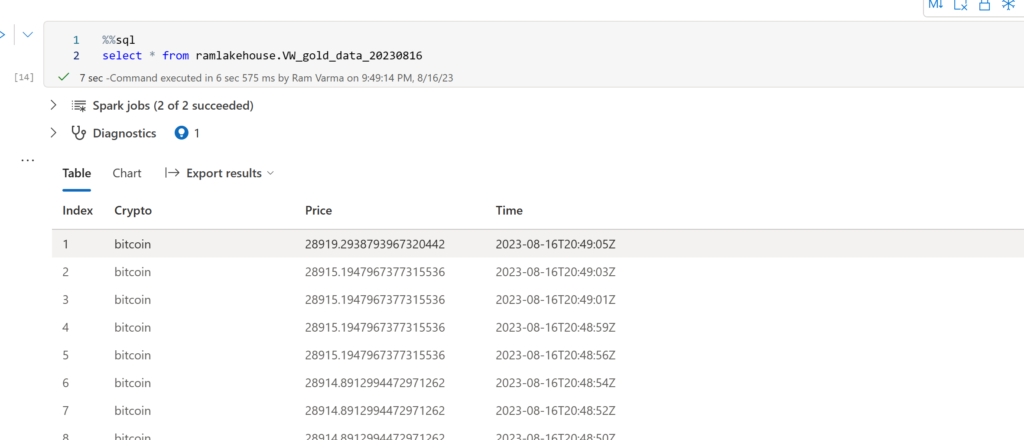
Tip from fabric: Optimize delta table with small file compaction.

Hope this would help your business to deal with real data and take your insights to next level .
Happy learning. Thank You!